自己紹介・研究目的
令和5年度入学/ ■SPRING事業 採択学生紹介
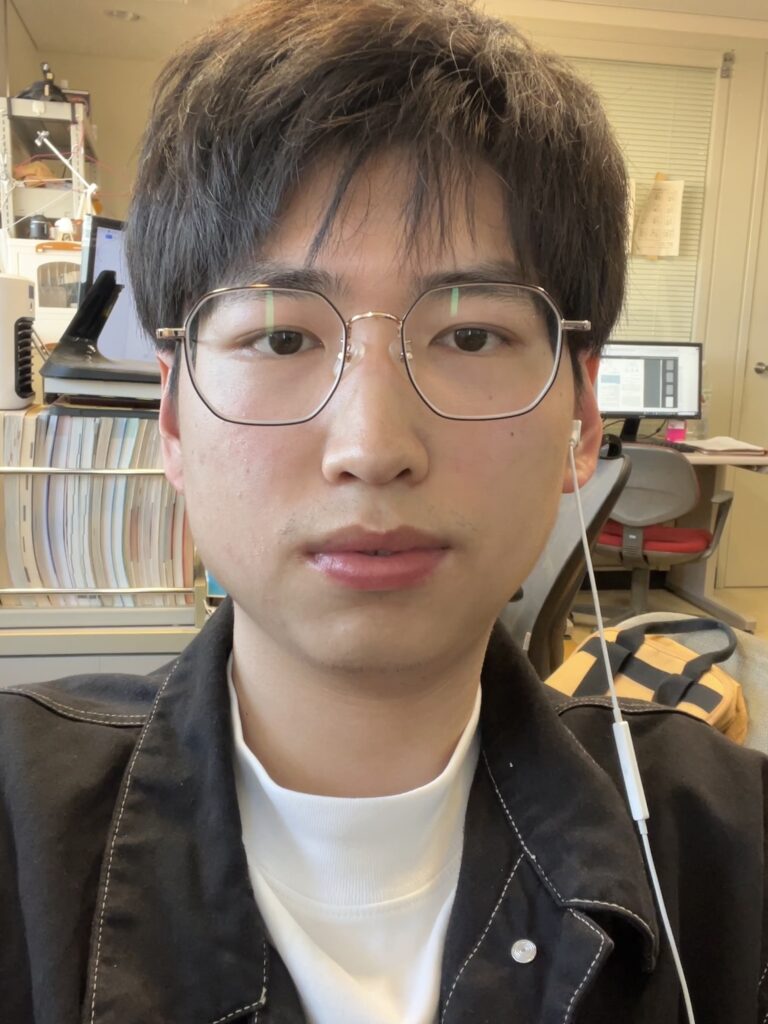
数理・ヒューマンシステム科学専攻
令和5年度 大学院入学
鄭 涛
テイ トウ
A Novel Evolutionary Wide Dendritic Learning
Hello, everyone. My name is Zheng Tao. I am a PhD student from the Artificial Intelligence Laboratory at the University of Toyama. My research interests primarily include evolutionary computing and neural networks. I hope to apply evolutionary computing and neural networks to solve real-world engineering problems and contribute to the development of humanity.
In recent years, deep learning (DL) has dominated the research area of artificial intelligence (AI) and achieved dramatic successes in speech recognition, images and videos processing, drug discovery, protein structure prediction, etc. To alleviate the inherent issues in DL, I plan to propose an Evolutionary Wide Dendritic Learning (EWDL) by systematically establishing its construction and learning methods and verifying its applications. The main purpose is enabling EWDL to be one of the next-generation interpretable and flexible learning techniques. The establishment of EWDL, which is an evolving network of DNM, will have significant scientific contributions to the AI community, including: 1) EWDL can be expected to significantly enhance the computational capacity of DL because a single DNM is much powerful than MCP. 2) As the information processing capacity of single neuron model has been significantly improved in DNM, the entire model complexity of EWDL can be greatly reduced. 3) EWDL only requires low computational energy consumption. 4) EWDL is much more interpretable than DL from the perspective of neuroscience.
(旧フェローシップ事業採択学生)
In recent years, deep learning (DL) has dominated the research area of artificial intelligence (AI) and achieved dramatic successes in speech recognition, images and videos processing, drug discovery, protein structure prediction, etc. To alleviate the inherent issues in DL, I plan to propose an Evolutionary Wide Dendritic Learning (EWDL) by systematically establishing its construction and learning methods and verifying its applications. The main purpose is enabling EWDL to be one of the next-generation interpretable and flexible learning techniques. The establishment of EWDL, which is an evolving network of DNM, will have significant scientific contributions to the AI community, including: 1) EWDL can be expected to significantly enhance the computational capacity of DL because a single DNM is much powerful than MCP. 2) As the information processing capacity of single neuron model has been significantly improved in DNM, the entire model complexity of EWDL can be greatly reduced. 3) EWDL only requires low computational energy consumption. 4) EWDL is much more interpretable than DL from the perspective of neuroscience.
(旧フェローシップ事業採択学生)
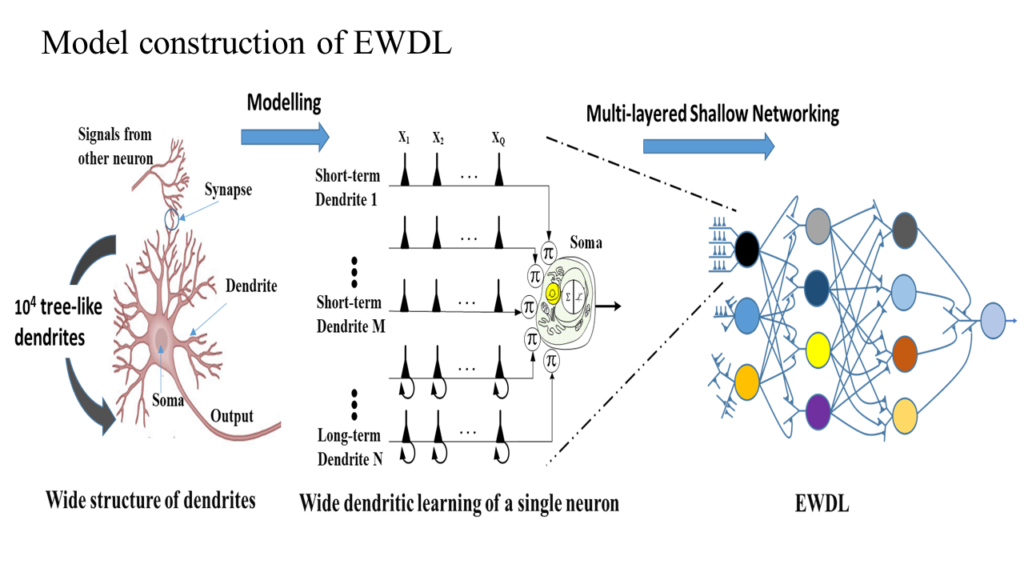