自己紹介・研究目的
令和4年度 支援終了学生修了/ ■SPRING事業 採択学生紹介
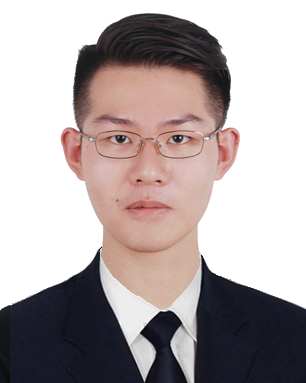
数理・ヒューマンシステム科学専攻
令和4年度 支援終了学生 大学院入学
張 祉明
チョウ シメイ
The Interpretability and Applied Research of Deep Learning
Hello everyone, my name is Zhiming Zhang (張 祉明). I am a Ph.D. in Advanced Mathematics and Human Mechanisms from Artificial Intelligence Lab. My research interests include machine learning, neural network, bioinformatics, and industrial informatics. I hope to contribute to solving real-world engineering and social problems through rigorous computer modeling, complete mathematical inference, and innovative approaches.
My research topic is The Interpretability and Applied Research of Deep Learning. I will explore how to make better use of deep learning to provide faster, more accurate, and lower-cost solutions to real-world problems. Compared with other deep learning methods, I will design a more specialized and interpretable approach closer to each industry's specific needs. I start my research from two real-world problems. The one is medical ultrasound imaging and the other is fluid dynamics prediction. In ultrasound imaging, a novel block-based sampling mechanism and fully-complex RNN are proposed to generate accurate ultrasound images. Compared with other methods, the proposed method in this work can greatly improve the speed and accuracy of medical ultrasound imaging. And in fluid dynamic prediction, a novel self-supervised-learning-based method is proposed to predict pressure by velocity field in turbulent flow. Because of the proposed self-supervised method, the deep network can find a most representative point in the complex velocity field as input data to make accurate predictions, which greatly reduces the difficulty of practical engineering. The two real-world problems solved above are very difficult problems in their respective fields. My proposed deep learning solutions significantly empower the development of both fields.(旧フェローシップ事業採択学生)
My research topic is The Interpretability and Applied Research of Deep Learning. I will explore how to make better use of deep learning to provide faster, more accurate, and lower-cost solutions to real-world problems. Compared with other deep learning methods, I will design a more specialized and interpretable approach closer to each industry's specific needs. I start my research from two real-world problems. The one is medical ultrasound imaging and the other is fluid dynamics prediction. In ultrasound imaging, a novel block-based sampling mechanism and fully-complex RNN are proposed to generate accurate ultrasound images. Compared with other methods, the proposed method in this work can greatly improve the speed and accuracy of medical ultrasound imaging. And in fluid dynamic prediction, a novel self-supervised-learning-based method is proposed to predict pressure by velocity field in turbulent flow. Because of the proposed self-supervised method, the deep network can find a most representative point in the complex velocity field as input data to make accurate predictions, which greatly reduces the difficulty of practical engineering. The two real-world problems solved above are very difficult problems in their respective fields. My proposed deep learning solutions significantly empower the development of both fields.(旧フェローシップ事業採択学生)
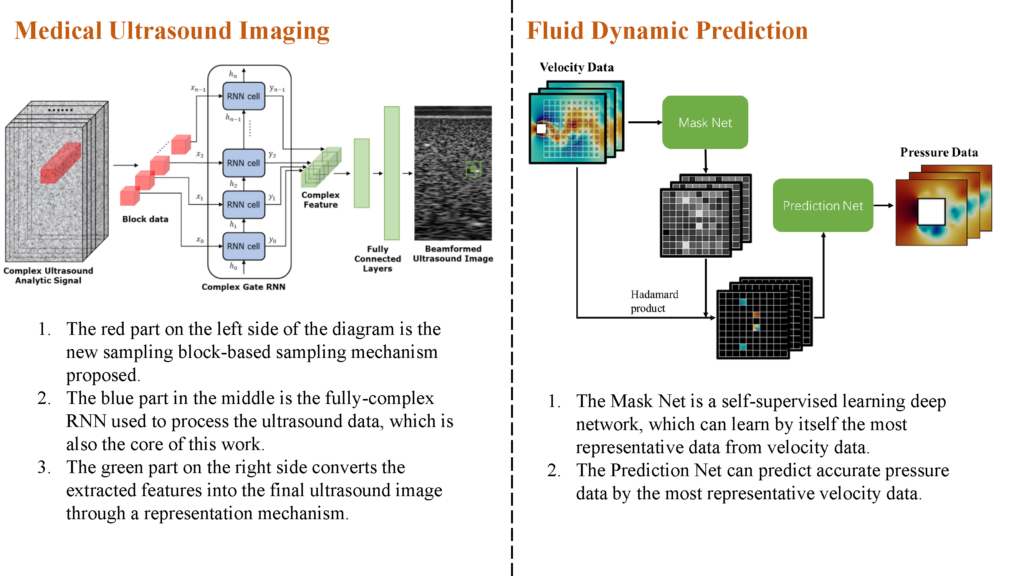