自己紹介・研究目的
令和5年4月入学/ ■SPRING事業 採択学生紹介
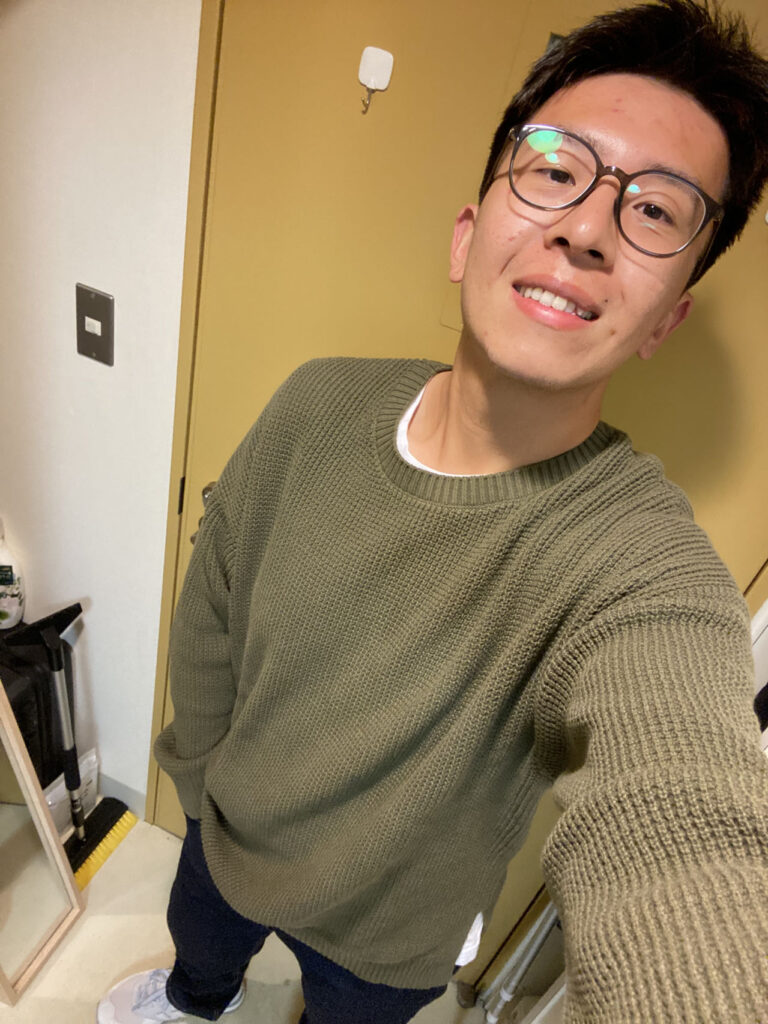
数理・ヒューマンシステム科学専攻
令和5年4月 大学院入学
張 宝航
チョウ ホウコウ
My Research on Evolutionary Algorithms: Past, Present, and Future
Hello, I'm Baohang Zhang, a first-year doctoral student at the Advanced Mathematics and Human Mechanisms, Artificial Intelligence Lab in the Graduate School of Science and Engineering, from Henan Province, China. My hobbies include singing, playing games, and traveling.
In my master's research, I focused on improving and designing evolutionary algorithms (EAs). EAs are optimization algorithms that produce better solutions through continuous evolution and optimization, based on the idea of natural evolution. I attempted to improve the Water Wave Optimization algorithm using multi-population mechanisms, improve the Whale Optimization algorithm using differential mechanisms, and used hybrid algorithms to compensate for their shortcomings. Before graduation, I proposed a new algorithm called the "Non-Revisiting Equilibrium Optimizer Algorithm" by combining non-repeating mechanisms.
Through two years of in-depth study of EAs, I have mastered many techniques to improve algorithm performance and gained deep thinking about EAs. To address practical problems, I plan to use feature selection (FS) problems as a starting point to broaden the application of EAs in my research.
Currently, my research direction is to use EAs to solve the feature selection problem (FS) in classification. Classification problems require dividing each sample in a dataset into one of several predetermined categories in machine learning. However, in practical applications, there are often irrelevant and redundant features in the dataset, which can reduce classification accuracy and increase computational costs. Therefore, FS has become a research focus and hotspot. To solve FS, many EAs have been introduced into classification problems. In FS problems, EAs can be used to search for the optimal feature subset to improve classification accuracy and efficiency.
I believe that the datasets used by the research community to measure the performance of EA-FS mostly come from practical problems, but there are still issues such as temporal bias and weak specificity. Therefore, my future research plan is to apply EA-FS in the field of new energy to solve practical problems.
(旧フェローシップ事業採択学生)
In my master's research, I focused on improving and designing evolutionary algorithms (EAs). EAs are optimization algorithms that produce better solutions through continuous evolution and optimization, based on the idea of natural evolution. I attempted to improve the Water Wave Optimization algorithm using multi-population mechanisms, improve the Whale Optimization algorithm using differential mechanisms, and used hybrid algorithms to compensate for their shortcomings. Before graduation, I proposed a new algorithm called the "Non-Revisiting Equilibrium Optimizer Algorithm" by combining non-repeating mechanisms.
Through two years of in-depth study of EAs, I have mastered many techniques to improve algorithm performance and gained deep thinking about EAs. To address practical problems, I plan to use feature selection (FS) problems as a starting point to broaden the application of EAs in my research.
Currently, my research direction is to use EAs to solve the feature selection problem (FS) in classification. Classification problems require dividing each sample in a dataset into one of several predetermined categories in machine learning. However, in practical applications, there are often irrelevant and redundant features in the dataset, which can reduce classification accuracy and increase computational costs. Therefore, FS has become a research focus and hotspot. To solve FS, many EAs have been introduced into classification problems. In FS problems, EAs can be used to search for the optimal feature subset to improve classification accuracy and efficiency.
I believe that the datasets used by the research community to measure the performance of EA-FS mostly come from practical problems, but there are still issues such as temporal bias and weak specificity. Therefore, my future research plan is to apply EA-FS in the field of new energy to solve practical problems.
(旧フェローシップ事業採択学生)
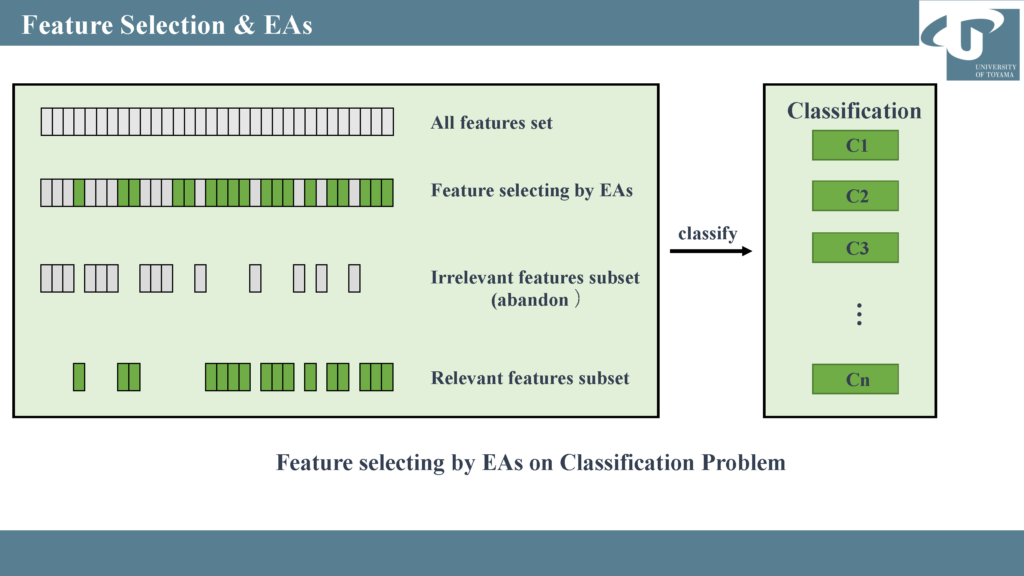