自己紹介・研究目的
令和5年度修了/ ■SPRING事業 採択学生紹介
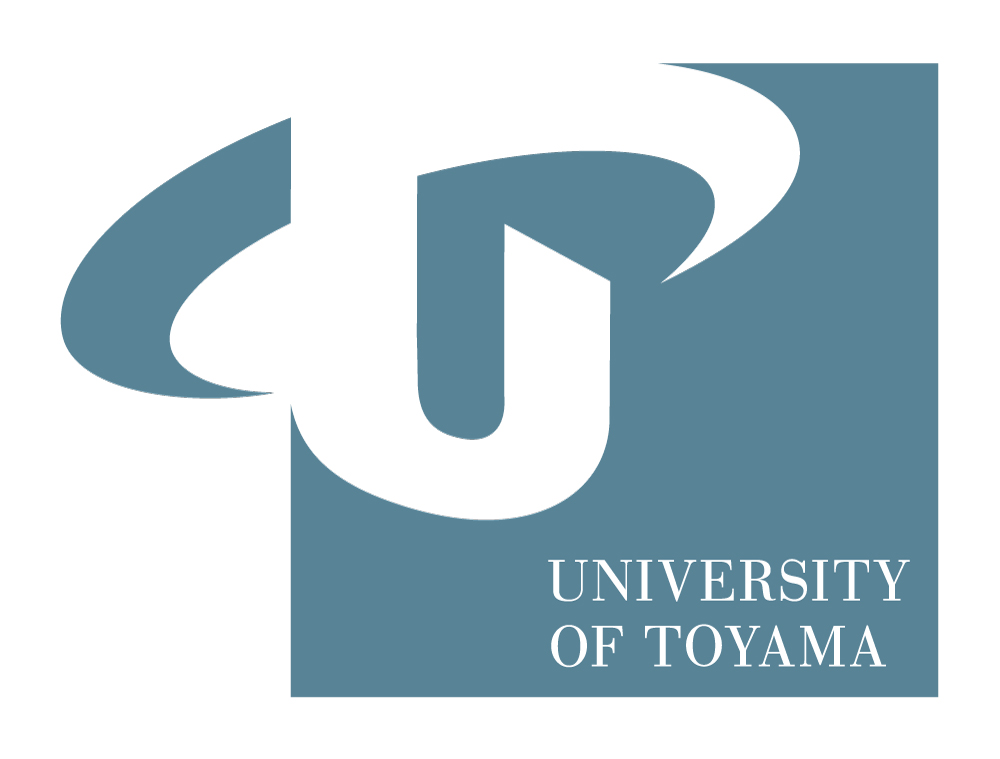
数理・ヒューマンシステム科学専攻
令和5年度 大学院入学
李 倩倩
リ センセン
A Machine Learning-based Model for Green House Data Prediction
Hello everyone, it is my honor to introduce myself here. My name is Qianqian Li, and I am a second-year doctor student in the University of Toyama. I am in the department of Advanced Mathematics and Human Mechanisms.
The greenhouse is used to protect crops from the outside environment. One of methods that to increase the quality and productivity of crops is creating the most suitable environment for crop growth.
The aim of my research is using the machine learning-based model to predict Time-serial data of temperature, humidity and Co2. My past research was focus on training hybrid Algorithm to solve benchmark problems; the research in the present is facing using algorithms in order to solve the real-word problems. Due to the population of AI in the recent years, there have been many publications on predicting the climatic conditions inside greenhouse. Comparing with these publications, my research prefers the accuracy of predictions. Accurate forecasting can help improve crop yields and quality. It is a great help for experimental fields as well. The human resources required in a greenhouse can be reasonably reduced, and it can be easier to provide the required environment for the test plots.
The greenhouse is used to protect crops from the outside environment. One of methods that to increase the quality and productivity of crops is creating the most suitable environment for crop growth.
The aim of my research is using the machine learning-based model to predict Time-serial data of temperature, humidity and Co2. My past research was focus on training hybrid Algorithm to solve benchmark problems; the research in the present is facing using algorithms in order to solve the real-word problems. Due to the population of AI in the recent years, there have been many publications on predicting the climatic conditions inside greenhouse. Comparing with these publications, my research prefers the accuracy of predictions. Accurate forecasting can help improve crop yields and quality. It is a great help for experimental fields as well. The human resources required in a greenhouse can be reasonably reduced, and it can be easier to provide the required environment for the test plots.
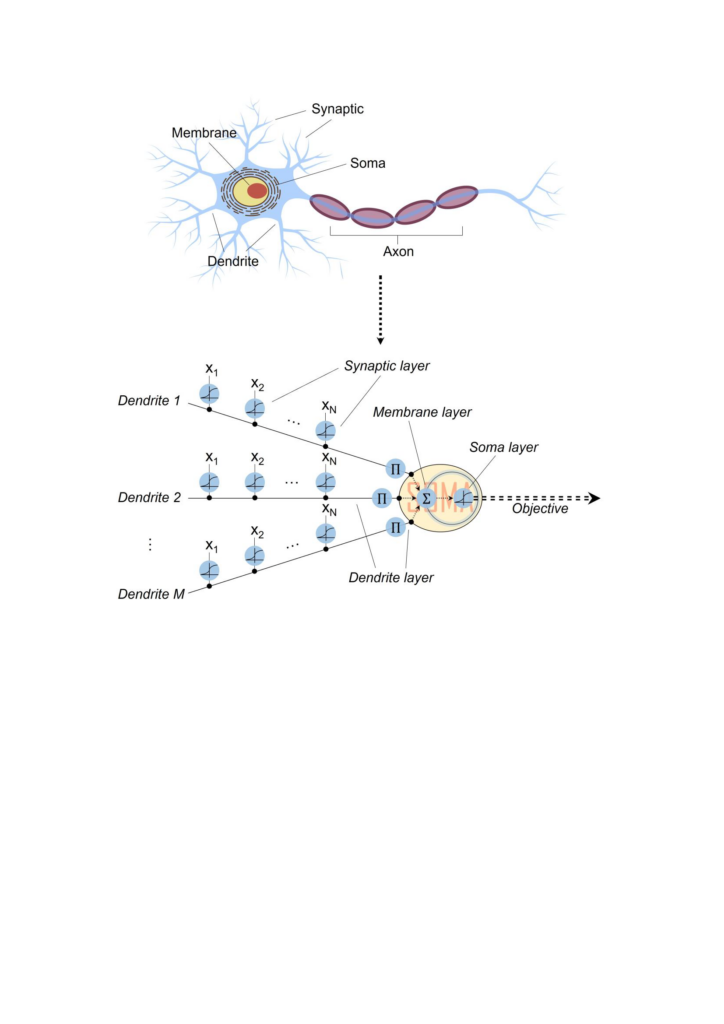